On the first week of being (mostly) off the grid, my only research was writing in the MCMC manual that Foreman-Mackey and I are writing. It is not as close to being done as I remembered it from way back (I think we started it in 2012).
2014-12-26
2014-12-19
bad chemical tags, earning travel
It was a day packed with non-research, except for group meeting, which was great, as always. Sanderson updated us on extensions of her action-space clustering methods for measuring the Milky Way gravitational potential. One of the ideas that emerged in the discussion relates to "extended distribution functions": In principle any stellar "tags" or labels or parameters that correlate with substructure identification could help in finding or constraining potential parameters. Even very noisy chemical-abundance labels might in principle help a lot. That's worth checking. Also, chemical labels that have serious systematics are not necessarily worse than labels that are "good" in an absolute sense. That is one of my great hopes: That we don't need good models of stars to do things very similar to chemical tagging.
Also in group meeting we gave Hattori marching orders for (a) getting a final grade on his independent study and then (b) getting to fly to Hawaii for the exoplanet stats meeting. For the former, he needs to complete a rudimentary search of all stars in Kepler. For the latter he needs to do hypothesis tests on the outcome of the search and write it up!
2014-12-17
calibration and search in Kepler; replacing humans
In group meeting we went through the figures for Dun Wang's paper on pixel-level self-calibration of Kepler, figure by figure. We gave him lots of to-do items to tweak the figures. This is all in preparation not just for his paper but also for AAS 225, which is in early January. At the end we asked "Does this set of figures tell the whole story?" and Fadely said "no, they don't show general improvement across a wide range of stars". So we set Wang on finding a set of stars to serve as a statistical "testbed" for the method.
Also in group meeting, Foreman-Mackey showed some of the systems returned by his search for long-period planets in the light-curves of bright Kepler G dwarf stars. So far it looks like he doesn't have anything that Kepler doesn't have, but he also thinks that some of the Kepler objects might be false positives.
We spent some time looking at Huppenkothen's attempt to reproduce human classification of states of black hole GRS 1915 using machine learning. We scoped a project in which she finds the features that do the best job of reproducing the human classification and then does an unsupervised clustering using those features. That should do something similar to the humans but possibly much better. She has good evidence that the unsupervised clustering will lead to changes in classes and classifications.
2014-12-16
job season
Research ground to a halt today in the face of job applications. There's always tomorrow.
2014-12-15
improving photometry hierarchically
Fadely handed me a draft manuscript which I expected to be about star–galaxy classification but ended up being about all the photometric measurements ever! He proposes that we can improve the photometry of an individual object in some band using all the observations we have about all other objects (and the object itself but in different bands). This would all be very model-dependent, but he proposes that we build a flexible model of the underlying distribution with hierarchical Bayes. We spent time today discussing what the underlying assumptions of such a project would be. He already has some impressive results that suggest that hierarchical inference is worth some huge amount of observing time: That is, the signal-to-noise ratios or precisions of individual object measurements rise when the photometry is improved by hierarchical modeling. Awesome!
Fadely and I also discussed with Vakili and Foreman-Mackey Vakili's project of inferring the spatially varying point-spread function in large survey data sets. He wants to do the inference by shifting the model and not shifting (interpolating or smoothing) the data. That's noble; we wrote the equations on the board. It looks a tiny bit daunting, but there are many precedents in the machine-learning literature (things like convolutional dictionary methods).
2014-12-14
git trouble
I spent a bit of Sunday working on paper one from The Cannon. Unfortunately, most of my time was spent resolving (and getting mad about) git fails, where my co-authors (who shall remain nameless) were editing wrong versions and then patching them in. Argh.
2014-12-12
redshift probability, lensed supernova, interstellar metallicity
At group meeting, Alex Malz showed some first results on using redshift probability distributions in a (say) luminosity function analysis. He showed that he gets different results if he takes the mean of the redshift pdf or the mode or does something better than either of those. I asked him to write that up so we can see if we all agree what "better" is. Fadely handed me a draft of his work to date on the star–galaxy separation stuff he has been working on.
After group meeting, at journal club, Or Graur (NYU) showed work he has been doing on a multiply imaged supernova. It looks very exciting, and it is multiply imaged by a galaxy in a lensing cluster, so there are actually something like seven or eight possibly detectable images of the supernova, some possibly with substantial time delays. Very cool.
The astro seminar was by Christy Tremonti (Wisconsin), who told us about gas and metallicity in galaxy disks. She has some possible evidence—in the form of gradients in effective yield and gas-to-star ratio—that the gas is being moved around the galaxy by galactic fountains. She is one of the first users of the SDSS-IV MaNGA integral-field data, so that was particularly fun to see.
2014-12-10
dotastronomy, day 3
The day started with the reporting back of results from the Hack Day. There were many extremely impressive hacks. The stand-outs for me—and this is a very incomplete list—were the following: Angus and Foreman-Mackey delivered two Kepler sonification hacks. In the first, they put Kepler lightcurves into an online sequencer so the user can build rhythms out of noises made by the stars. In the second, they reconstructed a pop song (Rick Astley, of course) using lightcurves as fundamental basis vectors. This just absolutely rocked. Along similar lines, Sascha Ishikawa (Adler) made a rockin' club hit out of Kepler lightcurves. Iva Momcheva did a very nice analysis of NASA ADS to learn things about who drops out of astronomy post-PhD, and when. This was a serious piece of stats and visualization work, executed in one day. Jonathan Fay (Microsoft) implemented the Astrometry.net API to get amateur photographs incorporated into World-Wide Telescope. Jonathan Sick (Queens) and Adam Becker (freelance) built tools to make context-rich bibliographic and citation information that could be used to build better network analysis of the literature. Stuart Lynn (Adler) augmented HTML with tags that are appropriate for fine-grained markup for scientific publications, with the goal of making responsive design for the scientific literature while preserving scholarly information and referencing. Hanno Rein (Toronto) built a realistic three-dimensional mobile-platform fly-through system for the HST 3D survey.
After these hacks, there were some great talks. The highlights for me included Laura Whyte (Adler) talking about their incredibly rich and deep programs for getting girls and under-represented groups to come in and engage deeply at Adler. Amazingly well thought out and executed. Stefano Meschiari (UT) blew us away with a discussion of astronomy games, including especially "minimum viable games" like Super Planet Crash, which is just very addictive. He has many new projects and funding to boot. He had thoughtful things to say about how games interact with educational goals.
Unconference proceeded in the afternoon, but I spent time recuperating, and discussing data analysis with Kelle Cruz (CUNY) and Foreman-Mackey.
2014-12-09
dotastronomy, day 2
Today was the Hack Day at dotastronomy. An incredible number of pitches started the day. I pitched using webcam images (behind a fisheye lens) from the Liverpool telescope on the Canary Islands to measure the sidereal day, the aberration of starlight, and maybe even things like precession and nutation of the equinoxes.
I spent much of the day discussing and commenting on other hacks: I helped a tiny bit with Angus and Foreman-Mackey's hack to sonify Kepler data, I listened to Jonathan Fay (Microsoft) as he complained about the (undocumented, confusing) Astrometry.net API, and I discussed testing environments for science with Arfon Smith (github) and Foreman-Mackey and others.
Very late in the evening, I decided to get serious on the webcam stuff. There is an image every minute from the camera and yet I found that I was able to measure sidereal time differences to better than a second, in any pair of images. Therefore, I think I have abundant precision and signal-to-noise to make this hack work. I went to bed having satisfied myself that I can determine the sidereal period, which is equivalent to figuring out from one day's rotation how many days there are in the year. Although I measured the sidereal day to nearly one part in 100,000, my result is equivalent to a within-a-single-day estimate for the length of the year of 366.6 days. If I use more than one image pair, or span more than one day in time, I will do far, far better on this!
2014-12-08
dotastronomy, day 1
Today was the first day of dotastronomy, hosted by the Adler Planetarium. There were talks by Arfon Smith (Github), Erin Braswell (Open Science), Dustin Lang (Astrometry), and Alberto Pepe (Authorea). Smith made a lot of parallels between the open collaborations built around github and scientific collaborations. I think this analogy might be deep. In the afternoon, unconference was characteristically diverse and interesting. Highlights for me included a session on making scientific articles readable on many platforms, and the attendant implications for libraries, journals, and the future of publishing. Also, there was a session on the putative future Open Source Sky Survey, for which Lang and I own a domain, and for which Astrometry.net is a fundamental technology (and possibly Enhance!). There were many good ideas for defining the mission and building the communities for this project.
At coffee break, Foreman-Mackey and I looked at McFee's project of using Kepler light-curves as basis vectors for synthesizing arbitrary music recordings. Late at night, tomorrow's hack day started early, with informal pitches and exploratory work at the bar. More on all this tomorrow!
2014-12-07
introduction and discussion
I hacked on Ness and my paper on data-driven models for stellar spectra.
2014-12-05
Kepler, uncertainties, inference
Bernhard Schölkopf showed up for the day today. He spent the morning working with Foreman-Mackey on search and the afternoon working with Wang on self-calibration of Kepler. In the latter conversation, we hypothesized that we might improve Wang's results if we augment the list of pixels he uses as predictors (features) with a set of smooth Fourier modes. This permits the model to capture long-term variability without amplifying feature noise.
Before that, in group meeting, Sanderson told us about the problem of assigning errors or uncertainties to our best-fit potential in her method for Milky Way gravitational potential determination. She disagrees with the referee and I agree with the referee. Ultimately, we concluded that the referee (and I) are talking about precision, but the accuracy of the method is lower than the precision. I think we understand why; it has something to do with the amount of structure (or number of structures) in phase space.
At lunch, we met up with David Blei (Columbia) and shot the shih about data science and statistics. Blei is a probabilistic inference master; we convinced him that he should apply his super-powers towards astrophysics. He offered one of our party a postdoc, on the spot!
2014-12-03
black holes and weird pixel effects
In group meeting, Huppenkothen argued out the projects we discussed on Monday related to machine classification of black-hole accretion states of GRS 1915. We talked about all three levels of project: Using supervised methods to transfer classifications for a couple of years of data onto all the other years of data, using unsupervised methods to find out how many classes there plausibly are for the state, and building some kind of generative model either for state transitions or for literally the time-domain photon data. We discussed feature selection for the first and second projects.
Also at group meeting, Foreman-Mackey showed a new Earth-like exoplanet he has discovered in the Kepler data! Time to open our new Twitter (tm) account. He also showed that a lot of his false positives relate to un-discovered discontinuities in the Kepler photometry of stars. After lunch, we spent time investigating these and building (hacky, heuristic) code to find them.
Here are the symptoms of these events (which are sometimes called "sudden pixel sensitivity drops"): They are very fast (within one half-hour data point) changes to the brightness of the star. Although the star brightness drops, in detail if you look at the pixel level, some pixels brighten and some get fainter at the same time. These events appear to have signs and amplitudes that are consistent with a sudden change in telescope pointing. However, they are not shared by all stars on the focal plane, or even on the CCD. Insane! It is like just a few stars jump all at once, and nothing else does. I am confused.
Anyway, we now have code to find these and (in our usual style) split the data at their locations.
2014-12-02
finding tiny planets, Kepler jumps, papers
Foreman-Mackey and I had a long and wide-ranging conversation about exoplanet search. He has search completeness in regimes of exoplanet period heretofore unexplored, and more completeness at small radii than anyone previously, as far as we can tell. However, his search still isn't as sensitive as we would like. We are doing lots of hacky and heuristic things ("ad hockery" as Jaynes and Rix both like to say), so there is definitely room for improvement. All that said, we might find a bunch of smaller and longer-period planets than anyone before. I am so stoked.
In related news, we looked at a Kepler star that suffered an instantaneous change in brightness. We went back to the pixel level, and found the discontinuity exists in every pixel in the star's image, but the discontinuity has different amplitudes including different signs in the different pixels. It is supposed to be some kind of CCD defect, but it is as if the star jumped in position (but its fellow stars on the CCD didn't). It is just so odd. When you do photometry at this level of precision, so many crazy thing appear.
Late in the day I caught up on reading and commenting on papers people have sent me, including a nice paper by Megan Shabram (PSU) et al on a hierarchical Bayesian model for exoplanet eccentricities, a draft paper (with my name on it) by Jessi Cisewski (CMU) et al on likelihood-free inference for the initial mass function of stars, a draft paper by Dustin Lang (CMU) and myself on principled probabilistic source detection, a paper by John Jenkins (Ames) et al on optimal photometry from a jittery spacecraft (think Kepler), and the draft paper by Melissa Ness et al on The Cannon.
2014-12-01
classification of black-hole states
I had a discussion today with Huppenkothen about the qualitatively different states of accreting black-hole GRS 1915. The behavior of the star has been classified into some dozen-ish different states, based on time behavior and spectral properties. We figured out at least three interesting approaches. The first is to do old-school (meaning, normal) machine learning, based on a training set of classified time periods, and try to classify all the unclassified periods. It would be interesting to find out what features are most informative, and whether or not there are any classes that the machine has trouble with; these would be candidates for deletion.
The second approach is to do old-school (meaning, normal) clustering, and see if the clusters correspond to the known states, or whether it splits some and merges others. This would generate candidates for deletion or addition. It also might give us some ideas about whether the states are really discrete or whether there is a continuum of intermediate states.
The third approach is to try to build a generative model of the path the system takes through states, using a markov model or something similar. This might reveal patterns of state switches. It could even work at a lower level and try to predict the detailed time-domain behavior, which is incredibly rich and odd. This is a great set of projects, and easy (at least to get started).
2014-11-30
magnetron
Over the Thanksgiving break, my only research was providing some comments to Huppenkothen on her paper (in prep) about fitting flexible models to magnetar bursts.
2014-11-25
spin-orbit and spin-spin in GR
Michael Kesden (UT Dallas) gave a great talk today about black-hole–black-hole binary orbits, and the spin–orbit and spin–spin interactions during inspiral. He showed that he can reduce the dimensionality of the spin interaction problem massively using invariants of the motion, and then obtained a regular, analytic solution for the spin evolution. This new solution will massively speed up post-Newtonian calculations of orbits and gravitational-wave waveforms and also will deliver new insight about relativistic dynamics. Along those lines, he found new resonances (spin-evolution resonances with the orbit) and three qualitatively different behaviors of the spin vectors in three different regimes of spin magnitude and alignment. It is also very related to everything I am teaching this semester!
2014-11-24
asteroseismology with Gaussian processes
After a low-research morning, Foreman-Mackey and I retreated to an undisclosed location to work on asteroseismology. We checked (by doing a set of likelihood evaluations) on behalf of Eric Agol (UW) whether there is any chance of measuring asteroseismological modes using a bespoke Gaussian process which corresponds to narrow Gaussians in the Fourier domain. The prospects are good (it seems to work) but the method seemed to degrade with signal-to-noise and sampling worse than I expected. Okay, enough playing around! Back to work.
2014-11-23
new capabilities for Kepler and TESS
I worked a bit today on building new capabilities for Kepler and TESS and everything to follow: In one project, we are imagining getting parallax information about stars in Kepler. This has been tried before, and there are many who have foundered on the rocks. We (meaning Foreman-Mackey and I) have a new approach: Let's, on top of a very flexible light-curve model, permit a term proportional to the sine and the cosine of the parallactic angle. Then let's consider the amplitude-squared of those coefficients as something that indicates the parallax. The idea is similar to that of the "reduced proper motion" method for getting distances: No proper motion is a parallax, but closer stars tend to have higher proper motions, so there is work that can be done with them. There the stochastic component is the velocity distribution in the disk. Here the stochastic component is the unmodeled flat-field variations.
In the other project I worked on today, I figured out how we might switch asteroseismology from it's current mode (take data to periodogram, take periodogram to measurements of mode frequencies and amplitudes; take mode frequencies to big and small differences; do science on the frequency differences) to one in which the most important quantity—the big frequency difference—is observed more-or-less directly in the data. I have a method, based on Gaussian Processes and Fourier transforms that I think might possibly work. One cool thing is that it might just might enable asteroseismology measurements on dwarf stars even in Kepler long-cadence data. That would be insane. Both of these projects are also great projects for TESS of course.
2014-11-21
exoplanet blast
Dave Charbonneau (Harvard) was in town today, to give the Big Apple Colloquium, which he did with flair. He emphasized the importance of exoplanets in any study of astrophysics or in any astrophysics group or department. He emphasized the amazing value of M-dwarfs as exoplanet hosts: Exoplanets are abundant around M-dwarfs, they are easier to detect there than around Sun-like stars, they are shorter-period at same temperature, and they tend to host small, rocky planets. He showed beautiful work (with Dressing) on the population statistics and also on the compositions of the small planets, which do indeed seem like they are Earth and Venus-like in their compositions. He also talked about TESS and it reminded me that the projects we have going here on Kepler and K2 should all also be pointed at TESS. It is often said that TESS will not be good for long-period planets, but in fact there is a large part of the sky that is viewed for the full year.
In group meeting we talked more about our crazy asteroseismology ideas. Foreman-Mackey and Fadely explained a recent paper about building Gaussian Processes with kernels that represent power spectra that are mixtures of Gaussians in Fourier space. I have a hope that we can use a model like this to do asteroseismology at very low signal-to-noise, bad cadence, and with massive missing data. Any takers?
In our morning informal talk, Yossi Shvartzvald (TAU) showed us results from a global network of planet-oriented microlensing monitor telescopes. They are observing so many stars in the bulge that there are some 50 events going off at all times in their data, and they are getting a few exoplanet discoveries per year. Awesome! He showed that we can already do some pretty confident planet occurrence rate calculations with the data, and this is the first time that we can constrain the statistics of true Jupiter and Saturn and Uranus analogs: Unlike RV and transits, the discoveries don't require monitoring for decades! Also, he talked about what is called "microlensing parallax", which is something I have been interested in for years, because it so beautifully converts angles into distances.
2014-11-19
ExoLab-CampHogg hack day
John Johnson (Harvard) came to NYU today along with a big fraction of his group: Ben Montet, Ruth Angus, Andrew Vanderburg, Yutong Shan. In addition, Fabienne Bastien (PSU), Ian Czekala (Harvard), Boris Leistedt (UCL), and Tim Morton (Princeton) showed up. We pitched early in the day, in the NYU CDS Studio Space, and then hacked all day. Projects included: Doing the occurrence rate stuff we do for planets but for eclipsing binaries, generalizing the Bastien "flicker" method for getting surface gravities for K2 data, building a focal-plane model for K2 to improve lightcurve extraction, documenting and build-testing code, modeling stellar variability using a mixture of Gaussians in Fourier space, and more! Great progress was made, especially on K2 flicker and focal-plane modeling. I very much hope this is the start of a beautiful relationship between our groups.
I also had long conversations with Leistedt about near-future probabilistic approaches to cosmology using our new technologies, Sanderson about series expansions of potentials for Milky Way modeling, Huppenkothen about AstroHackWeek 2015, and Vakili about star centroiding. In somewhat related news, during the morning pitch session, I couldn't stop myself from describing the relationships I see between structured signals, correlation functions, power spectra, Gaussian processes, cosmology, and stellar asteroseismology. I think we might be able to make asteroseismology more productive with smaller data sets.
2014-11-18
AAAC, day 2
In the second day, we heard from Ulvestad (NSF) about how budgets are planned at the agencies, from the presidential request through to the divisions and then adjusted over time. It was remarkable and scary! Although the granting is all peer-reviewed, there is a huge amount of decision-making within NSF that is certainly not. That said, astronomy does well at NSF in part because it has well-organized, unified community support for certain big projects.
We spent a long time talking about principles for access to shared facilities and federally funded observatories and surveys and other such projects. One principal principle is transparency, which I love. Another is open data. We also spent a lot of time talking about the possible data we would need to understand the causes of (and solutions to) the related problems of low success rates on grant proposals and the large number of proposals submitted per person per year.
2014-11-17
AAAC, day 1
Today was the first day of the Astronomy and Astrophysics Advisory Committee meeting at NSF headquarters. The Committee is established by an act of Congress to oversee the interagency cooperation and interaction and etc between NSF Astronomy and Astrophysics and NASA Astrophysics (and also DOE Cosmic Frontiers). I learned a huge amount about science at the meeting, including about a conflict between VLBI and Hipparcos parallaxes to the Pleaides. That's Huge. Of course we looked at the outrageously awesome ALMA image of HL Tau showing actual Oh-My-God rings. I learned that the black hole at the center of M82 is no longer thought to be a black hole (need to learn more about that!) and that there is a too-massive black hole found at an ultra-compact dwarf galaxy. Wow, science rocks!
We went on to learn that science rocks a lot less than I thought, for various reasons: The proposal success rates in most individual-investigator money grants are at 15 to 20 percent, with DOE being higher but with most of their (DOE's) grants going to groups already working on DOE-priority projects. These low success rates may be distorting the "game" of applying for funding; indeed it appears that proposers are writing more proposals per year than ever before.
I learned (or re-learned) that the federal budgets (primarily from the executive branch) that involve ramping down work on NASA SOFIA are also budgets that involve ramping down the whole NASA Astrophysics budget by the same amount. That is, the honesty of the community and its willingness to make hard choices about what's important leads to budget reductions. Those are some terrible incentives being set up for the community. The agencies and the powers that be above them are creating a world in which honesty and frugality is rewarded with budget cuts. I guess that's why the defense part of the US government is so (a) large and (b) dishonest. Thanks, executive branch! Okay, enough goddamn politics.
2014-11-16
grant proposal
I spent the day working finishing my grant proposal to the NSF (due tomorrow). It is about probabilistic approaches to cosmology. In the end, I am very excited about the things that can be enabled if we can find ways to make a generative model of things like the positions of all galaxies or the unlensed shapes of galaxies: We can connect the theoretical model (which generates two-point functions of primordial density fields) to the data (which are positions and shapes of galaxies, among other things). That can only increase our capabilities, with existing and future data.
2014-11-14
The Cannon hack week, day 5
A highlight of group meeting today was Sander Dieleman (Ghent) explaining to us how he won the kaggle Galaxy Zoo challenge. His blog post about it is great too. We hope to use something like his technology (which he also applies to music analysis) to Kepler light-curves. The convolutional trick is so sensible and clever I can't stand it. His presentation to my group reminds me that no use of deep learning or neural networks in astronomy that I know about so far has ever really been sophisticated. Of course it isn't easy!
Ness and I spent the full afternoon working on The Cannon, although a lot of what we talked about was context and next projects, which informs what we write in our introduction and discussion, and what we focus on for paper 2. One thing we batted around was: What kind of projects can we do with these labels that no-one has done previously? I was asking this because we have various assumptions about our "customer" and if our customer isn't us, we might be making some mistakes. Reminds me of things we talked about at DDD.
2014-11-12
The Cannon hack week, day 3
In my view, the introduction of a paper should contextualize, bring up, form, and ask questions, and the discussion section at the end should answer them, or fail to, or answer them with caveats. The discussion should say all the respects in which the answers given are or could be or shoud be wrong. We only understand things by understand what they don't do. (And no paper should have a "summary" section: Isn't that the abstract?) Ness and I assembled our conclusions, caveats, and questions about The Cannon into an outline for the discussion section. We also talked a bit more about figures. She spent the day working on discussion and I worked a bit on the abstract.
In group meeting, we looked at Malz's first models of SDSS sky. Foreman-Mackey brought up the deep question: If we have a flexible model for the sky in SDSS fibers, how can we fit or constrain it without fitting out or distorting the true spectra of the astronomical objects that share the fibers? Great question, and completely analogous to the problem being solved by Wang in our model for Kepler pixels: How do we separate the signals caused by the spacecraft from those caused by exoplanets?
We are approaching Wang's problem by capitalizing on the causal or conditional independence properties of our generative model. But this is imperfect, since there are hyper-priors and hyper-hyper-priors that make everything causally related to everything else, in some sense. One example in the case of sky: Atomic and molecular physics in the atmosphere is the same atomic and molecular physics acting in stellar atmospheres and the interstellar medium in the spectra of astronomical sources. Another example: The line-spread function in the spectrograph is the same for sky lines and for galaxy emission and absorption lines. These kinds of commonalities make the "statistically independent" components in fact very similar.
2014-11-11
The Cannon hack week, day 2
Our main progress on The Cannon, paper 1, was to go through all the outstanding discussion points, caveats, realizations, and notes, and use them to build an outline for a Discussion section at the end of the paper. We also looked at final figure details, like colors (I am against them; I still read papers printed out on a black-and-white printer!), point sizes, transparency, and so on. We discussed how to understand the leave-one-out cross-validation, and why the leave-one-star-out cross-validation looks so much better than the leave-one-cluster-out cross-validation: In the latter case, when you leave out a whole cluster, you lose a significant footprint in the stellar label-space in the training data. The Cannon's training data set is a good example of something where performance improves a lot faster than square-root of N.
2014-11-10
The Cannon hack week, day 1
Melissa Ness arrived in NYC for a week of hacking on The Cannon, our project to transfer stellar parameter labels from well-understood stars to new stars using a data-driven model of infrared stellar spectra from APOGEE. We discussed nomenclature, notation, figures, and the paper outline. The hope is to get a submittable draft ready by Friday. I am optimistic. There are so many things we can do in this framework, the big issue is limiting scope for paper 1.
One big important point of the project is that this is not typical machine learning: We are not transforming spectra into parameter estimates, we are building a generative model of spectra that is parameterized by the stellar parameter labels. This permits us to use the noise properties of the spectra that we know well, generalize from high signal-to-noise training data to low signal-to-noise test data, and account for missing and bad data. The second point is essential: In problems like this, the training data are always much better than the test data!
2014-11-09
probabilistic tools for cosmology
I worked on my NSF proposal today. I am trying to figure out how the different threads we have on cosmology tie together: We are working on density-field modeling with Gaussian Processes, hierarchical probabilistic models for weak lensing, and probabilistic models for the point-spread function. We also have quasar target selection in the far past and approximate Bayesian computation (tm) in the possible near future. I tried to weave some combination of these into a project summary but am still confused about the best sell.
2014-11-07
new exoplanet, black-hole states, and more
At group meeting, Hattori showed us an exoplanet discovery, made with his search for single transits! Actually, the object was a known single transit, but Hattori showed that it is in fact a double transit and has a period very different from its recorded value. So this counts as a discovery, in my book. We are nearly ready to fully launch Hattori's code "in the data center"; we just need to run it on a bunch more cases to complete our functional testing.
Also at group meeting, Sanderson discussed the Gaia Challenge meeting from the previous week. There are lots of simulated Gaia-like data sets available for testing methods and ideas for Gaia data analysis. This is exciting. We also discussed generalizing her structure-finding code to make it also a clustering algorithm on the stars.
Also at group meeting, Daniela Huppenkothen (NYU) showed us time-series and spectral data from the famous black-hole source GRS 1915, which has about a dozen different "states". She suggested that there might be lots of low-hanging fruit in supervised and unsupervised classification of these different states, using both time features and spectral features. The data are so awesome, they could launch a thousand data-science masters-student capstone projects!
Tsvi Piran (Racah) gave a lively talk on the likely influence of gamma-ray bursts on life on earth and other habitable worlds. He argued that perhaps we live so far out in the outskirts of the Milky Way because the GRB rate is higher closer to the center of the Galaxy, and GRBs hurt. The ozone layer, that is.
2014-11-06
cosmic origins
Short conversations today with Mei and Lang and Foreman-Mackey. Mei and I decided that we should do the continuum normalization that he is doing at the raw-data stage, before we interpolate the spectra onto the common wavelength grid. This should make the interpolation a bit safer, I think. Lang and I discussed DESI and he showed me some amazing images, plus image models from The Tractor. Foreman-Mackey and I discussed the relevance of his exoplanet research program to the NASA Origins program and the Great Observatories. Can anyone imagine why?
2014-11-05
the sky in spectroscopy
At the end of the day, Malz came by the CDS space and we talked through first steps on a project to look at sky subtraction in spectroscopy. We had a great idea: If the sky residuals are caused by small line-shape changes, we can model the sky in each fiber with a linear combination of other sky fibers, including those same fibers shifted left and right by one pixel. This is like the auto-regression we do for variable stars—and they do on Wall St to model price changes in securities—but applied in the wavelength direction. It ought to permit the sky fitting to fit out convolution (or light deconvolution) as well as the brightness.
Group meeting included some very nice plots from Fadely showing that he can model the color-size distribution of sources in the SDSS data, potentially very strongly improving star–galaxy separation. We also talked about ABC (tm), or Bayesian inference when you can't write down a likelihood function, and also stellar centroiding.
At lunch, Goodman told the Data Science community about affine-invariant MCMC samplers. He did a good job advertising emcee and some new projects he is working on.
2014-11-03
probabilistic cosmology
I spent time working through some inference problems in the future of cosmology, including how you use probabilistic redshift information, how you marginalize out beliefs about the density field and galaxy formation within that density field, and how you might get probabilistic information about the 2-pt function. I am confused about how much this all matters for different kinds of projects.
At lunch, Andy Haas (NYU) told us about searches for milli-charged particles at the LHC. He has a plan that would rule out a huge class of models.
2014-11-02
p(z)?
I learned from Brant Robertson (Arizona), on twitter (tm) of all places, that the LSST level-2 data products will include probabilistic information about source redshifts. I spent some time working out how a "LSST customer" might use those data products. Most of the simple ideas one might have (weight by p(z) at each redshift, for example) are dead wrong and will wrong-ify your answers rather than make them more accurate. Now I am thinking: NSF proposal?
2014-10-31
quasars! exoplanets! dark matter at small scales!
CampHogg group meeting was impressive today, with spontaneous appearances by Andreu Font-Ribera (LBL), Heather Knutson (Caltech), and Lucianne Walkowicz (Adler). All three told us something about their research. Font-Ribera showed a two-dimensional quasar—absorption cross-correlation, which in principle contains a huge amount of information about both large-scale structure and the illumination of the IGM. He seems to find that IGM illumination is simple or that the data are consistent with a direct relationship between IGM absorption and density.
Knutson showed us results from a study to see if stars hosting hot Jupiters on highly inclined (relative to the stellar rotation) orbits are different in their binary properties from those hosting hot Jupiters on co-planar orbits. The answer seems to be "no", although it does look like there is some difference between stars that host hot Jupiters and stars that don't. This all has implications for planet migration; it tends to push towards disk migration having a larger role.
We interviewed Walkowicz about the variability of the Sun (my loyal reader will recall that we loved her paper on the subject). She made a very interesting point for future study: The "plage" areas on the Sun (which are brighter than average) might be just as important as the sunspots (which are darker than average) in causing time variability. Also, the plage areas are very different from the sunspots in their emissivity properties, so they might really require a new kind of model. Time to call the applied mathematics team!
In the afternoon, Alyson Brooks (Rutgers) gave the astro seminar, on the various issues with CDM on small scales. Things sure have evolved since I was working in this area: She showed that the dynamical influence of baryonic physics (collapse, outflows, and so on) are either definitely or conceivably able to create the issues we see with galaxy density profiles at small scales, the numbers of visible satellites, the density distribution of satellites, and the sizes of disk-galaxy bulges. On the latter it still seems like there is a problem, but on the face of it, there is not really any strong reason to be unhappy with CDM. As my loyal reader knows, this makes me unhappy! How can CDM be the correct theory at all scales? All that said, Brooks herself is hopeful that precise tests of CDM at galaxy scales will reveal new physics and she is doing some of that work now. She also gave great shout-outs to Adi Zolotov.
2014-10-29
single transits, redshift likelihoods
A high research day today, for the first time in what feels like months! In group meeting in the finally-gutted NYU CDS studio space, So Hattori told us about some single transits in Kepler and his putative ability to find them. We unleashed some project management on him and now he has a great to-do list. No success in CampHogg goes unpunished! Along the way, he re-discovered a ridiculously odd Kepler target that has three transits from at least two different kinds of planets, neither of which seems periodic. Or maybe it is one planet around a binary host, or maybe worse? That launched some email trail with some Kepler peeps.
Also at group meeting, Dun Wang showed some near-final tests of the hyper-parameter choices in his data-driven model of the Kepler pixels. It is getting down to details, but details matter. We came up with one final possible simplification for his hyper-parameter choices for him to test this week.
In the afternoon, Alex Malz came by to discuss Spring courses and we ended up working through a menu of possible thesis projects. One that I pitched is so sweet: It is just to write down, very carefully, what we would do if we had instead of a redshift catalog a set of low-precision redshift likelihood functions (with SED or spectral nuisance parameters). Could we then get the luminosity function and spatial clustering of galaxies? Of course we could, but we would have to go hierarchical. Is this practical at LSST scale? Not sure yet.
2014-10-28
text, R, and politics
Today at lunch Michael Blanton organized a Data Science event in which Ken Benoit (LSE) told us about quanteda, his package for manipulating text in R. This package does lots of the data massaging and munging that used to be manual work, and gets the text data into "rectangular" form for data analysis. It also does lots of data analysis tasks too, but the munging was very interesting: Part of Benoit's motivation is to make text analyses reproducible from beginning to end. Benoit's example texts were amusing because he works on political speeches. He had examples from US and Irish politics. Some discussion in the room was about Python vs R; the key motivation for working in R is that it is by far the dominant language at the intersection of statistics and political science.
2014-10-27
nuclear composition of UHECRs
Today Michael Unger (Karlsruhe) told us over lunch about ultra-high energy cosmic rays from Auger. There are many mysteries, but it does look like the composition moves to higher-Z nuclei as you go to higher energies, or at least that's my read. He told us also about a very intriguing extension to Auger which would make it possible to distinguish protons from iron in the ground detectors; if that became possible, it might be possible to do cosmic-ray imaging: It is thought that the cosmic magnetic fields are small enough that protons near the GZK cutoff should point back to their sources. So far this hasn't been possible, presumably because the iron (and other heavy elements) have charge-to-momentum ratios too large; they get heavily deflected by the magnetic fields they encounter.
2014-10-26
Math-Astrophysics collaboration proposal
I spent a big chunk of the day today trying to write a draft of a collaboration proposal (really a letter of intent) for the Simons Foundation. That is only barely research.
2014-10-24
exoplanet compositions
Today Angie Wolfgang (UCSC) gave a short morning seminar about hierarchical inference of exoplanet compositions (like are they ice or gas or rock?). She showed that the super-Earth (1 to 4 Earth-radius) planet radius distribution fairly simply translates into a composition distribution, if you are willing to make the (pretty justified, actually) assumption that the planets are rocky cores with a hydrogen/helium envelope. She inferred the distribution of gas fractions for these presumed rocky planets and got some reasonable numbers. Nice! There is much more to do, of course, since she cut to a very clean sample, and hasn't yet looked at the interdependence of composition, period, and host-star properties. There is a lot to do in exoplanet populations still!
2014-10-22
training convolutional nets to find exoplanets
In group meeting today, a good discussion arose about training a supervised method to find exoplanet transits. Data-Science Masters student Elizabeth Lamm (NYU) is working with us to use a convolutional net (think: deep learning) to find exoplanet transits in the Kepler data. Our rough plan is to train this net using real Kepler lightcurves into which we have injected artificial planets. This will give "true positive" training examples, but we also need "true negative" examples. Since transits are rare, most of the lightcurves would make good negative training data; even if we used all of the non-injected lightcurves arbitrarily, we would only have a false-negative rate of a tiny fraction of a percent (like a hundredth of a percent).
That said, there were various intuitions (about training) represented in the discussion. One intuition is that even this low rate of false negatives might lead to some kinds of over-fitting. Another is that perhaps we should up-weight in the training data true negatives that are "threshold crossing events" or, in other words, places where simple software systems think there is a transit but close inspection says there isn't. We finished the discussion in disagreement, but realized that Lamm's project is pretty rich!
2014-10-21
K2 pointing model
Imagine a strange "game": A crazy telescope designer put thousands of tiny pixelized detectors in the focal plane of an otherwise stable telescope and put it in space. Each detector has an arbitary position in the focal plane, orientation, and pixel scale, or even non-square (affine) pixels. But given the stability, the telescope's properties are set only by three Euler angles. How can you build a model of this? Ben Montet (Harvard CfA), Foreman-Mackey, and I worked on this problem today. Our approach is to construct a three-dimensional "latent-variable" space in which the telescope "lives" and then an affine transformation for each detector patch. It worked like crazy on the K2 data, which are the data from the two-wheel era of the NASA Kepler satellite. Montet is very optimistic about our abilities to improve both K2 and Kepler photometry.
2014-10-20
single transits, new physics, K2
In my small amount of research time, I worked on the text for Hattori's paper on single transits in the Kepler data, including how we can search for them and what can be inferred from them. At lunch, Josh Ruderman (NYU) gave a nice talk on finding beyond-the-standard-model physics in the Atlas experiment at LHC. He made a nice argument at the beginning of his talk that there must be new physics for three reasons: baryogenesis, dark matter, and the hierarchy. The last is a naturalness argument, but the other two are pretty strong arguments! In the afternoon, while I ripped out furniture, Ben Montet (Harvard) and Foreman-Mackey worked on centroiding stars in the K2 data.
2014-10-17
three talks
Three great talks happened today. Two by Jason Kalirai (STScI) on WFIRST and the connection between white dwarf stars and their progenitors. One by Foreman-Mackey on the new paper on M-dwarf planetary system abundances by Ballard & Johnson. Kalirai did a good job of justifying the science case for WFIRST; it will do a huge survey at good angular resolution and great depth. He distinguished it nicely from Euclid. It also has a Guest Observer program. On the white-dwarf stuff he showed some mind-blowing color-magnitude diagrams; it is incredible how well calibrated HST is and how well Kalirai and his team can do crowded-field photometry, both at the bright end and at the faint end. Foreman-Mackey's journal-club talk convinced us that there is a huge amount to do in exoplanetary system population inference going forward; papers like Ballard & Johnson only barely scratch the surface of what we might be doing.
2014-10-16
regression of continuum-normalized spectra
I had a short phone call this morning with Jeffrey Mei (NYUAD) about his project to find the absorption lines associated with high-latitude, low-amplitude extinction. The plan is to do regression of A and F-star spectra against labels (in this case, H-delta EW as a temperature indicator and SFD extinction), just like the project with Melissa Ness (MPIA) (where the features are stellar parameters instead). Mei and I got waylaid by the SDSS calibration system, but now we are working on the raw data, and continuum-normalizing before we regress. This gets rid of almost all our calibration issues. The remaining problem (which I don't know how to solve) is the redshift or rest-frame problem: We want to work on the spectra in the rest frame of the ISM, which we don't know!
2014-10-15
measuring the positions of stars
At group meeting, Vakili showed his results on star positional measurements. We have several super-fast, approximate schemes that come close to saturating the Cramér–Rao bound, without requiring a good model of the point-spread function.
One of these methods is the (insane) method used in the SDSS pipelines, which was communicated to us in the form of code (since it isn't fully written up anywhere). This method (due to Lupton) is genius, fast, runs on minimal hardware with almost no overhead, and comes close to saturating the bound. Another of these is the method made up on the spot by Price-Whelan and me when we wrote this paper on digitization bandwidth, with a small modification (involving smoothing (gasp!) the image); the APW method is simpler and faster than the SDSS method on modern compute machinery.
Full-up PSF modeling should beat (very slightly) both of these methods, but it degrades in an unknown way as the PSF model gets wrong, and who is confident that he or she has a perfect PSF model? Vakili is going to have a nice paper on all this; we started writing it just as an aside to other things we are doing, but we realized that much of what we are learning is not really in the literature. Let's hear it for the analysis of astronomical engineering infrastructure!
2014-10-14
software and literature; convex problems
Fernando Perez (Berkeley), Karthik Ram (Berkeley), and Jake Vanderplas (UW) all descended on CampHogg today, and we were joined by Brian McFee (NYU) and Jennifer Hill (NYU) to discuss an idea hatched by Hill at Asilomar to build a system to scrape the literature—both refereed and informal—for software use. The idea is to build a network and a recommendation system and alt metrics and a search system for software in use in scientific projects. There are many different use cases if we can understand how papers made use of software. There was a lot of discussion of issues with scraping the literature, and then some hacking. This has only just begun.
At lunch, I visited the Simons Center for Data Analysis. I ended up having a long conversation with Christian Mueller (Simons) about the intersection of statistics with convex optimization. Among other things, he is working on principled methods for setting the hyperparameters in regularized optimizations. He told me many things I didn't know about convex problems in data analysis. In particular, he indicated that there might be some very clever and provably optimal (or non-sub-optimal) ways to reduce the feature space for the "Causal Pixel Model" for Kepler pixels that Wang is working on.
2014-10-10
Kepler occurrence rate review, day 2
Today the review committee wrote up and presented recommendations to the Kepler team on it's close-out planet occurrence rate inference plans. We recommended that the big issues in occurrence rate—especially near Earth-like planets—are factor-of-two and larger, so the team ought to focus on the big things and not spend time tracking down percent-level effects. After the review I had long talks with Jon Jenkins (Ames) and Tom Barclay (Ames) about Kepler projects and tools.
2014-10-09
Kepler occurrence rate review, day 1
Today I got up at dawn's crack and drove to Mountain View for a review of the NASA Kepler team's planet occurrence rate inferences. It was an incredible day of talks and conversations about the data products and experiments needed to turn Kepler's planet (or object-of-interest) catalog into a rate density for exoplanets, and especially the probabilities that stars host Earth-like planets. We spent time talking about high-level priorities, but also low-level methodologies, including MCMC for uncertainty propagation, adaptive experimental design for completeness (efficiency) estimation, and the relative merits of forward modeling and counting planets in bins. On the latter, the Kepler team is creating (and will release publicly) everything needed for either approach.
One thing that pleased me immensely is that Foreman-Mackey's paper on the abundance of Earth analogs got a lot of play in the meeting as an exemplar of good methodology, and also an exemplar of how uncertain we are about the planet occurrence rate! The Kepler team—and increasingly the whole astronomical community—is coming around to the view that forward modeling methods (as in hierarchical probabilistic modeling or approximate bayesian computation) are preferable to counting dots in bins.
2014-10-08
DSE Summit, day 3
On the last day of the Summit, we spent the full meeting talking about the collaboration and deliverables for the funding agencies. That does not qualify as research. Late in the day I had a revelation about the relationship between ethnography and science. They are related, but not really the same. Some of the conclusions of ethnography have a factual or hypothesis-generating character, but ethnographic results do not really live in the same domain as scientific results. That is no knock on ethnography! Ethnographers can ask questions that we don't even know how to start to ask quantitatively.
2014-10-07
DSE Summit, day 2
On the second day of the Moore–Sloan Data Science Summit, we did some awesome community building exercises involving team problem-solving. We then discussed and tried to understand how it relates to our ideas about collaboration and creativity. That was pretty fun!
At lunch I had a great conversation with Philip Stark (Berkeley) about finding signals in time series below the Nyquist (sampling) limit; in principle it is possible if you have a good idea what you are looking for or what's hidden there. We also talked about geometric descriptions of statistics: The world is infinite dimensional (there are a set of fields at every position in phase space) but observations are finite (noisy measurements of certain kinds of projections). This has lots of implications for the impact of priors (such as non-negativity), when they apply to the infinite-dimensional object (the latent variables, rather than the finite observations).
After lunch, it was probabilistic generalizations of periodograms with Jake Vanderplas (UW) and some frisbee, and then a discussion about the open spaces for Data Science that we are building at Berkeley, UW, and NYU. In all three, there are issues of setting the rules and culture of the space. I think the three institutions can make progress together that no one institution could make on its own.
2014-10-06
DSE Summit, day 1
Today was the first day of the community-building meeting of the Moore-Sloan Data Science Environments, held at Asilomar (near Monterey, CA). The project is a collaboration between Berkeley, UW Seattle, and NYU; the meeting has about 100 attendees from across the three institutions. The day started with an unconference in the morning, in which I attended a discussion session on text and text analysis. After that, we got into small inter-institutional groups and worked out our commonalities (and then presented them as lightning talks), as a way to get to know one another and also introduce ourselves to the community. Much of the community building happened on the beach!
2014-10-03
the Sun is normal; how did Jupiter form?
At group meeting, Wang reviewed Basri, Walkowicz, & Reiners (2013) on the variability of the Sun in terms of Kepler stars. It shows that (despite rumors to the contrary) the Sun is very typically variable for G-type dwarf stars. It is a very nice piece of work; it just shows summary statistics, but they are nicely robust and insensitive to satellite systematics.
Also in group meeting, Vakili showed first results from a dictionary-learning approach to the point-spread function in LSST simulated imaging. He is using stochastic gradient descent, which I learned (in the meeting) is useful for starting off an optimization, even in cases where the full likelihood (or objective) function can be computed just fine.
After lunch, Roman Rafikov (Princeton) gave a nice talk about the formation of giant planets. He argued that distant planets (like in HR 8799) might have a different formation mechanism that close planets (like Jupiter and hot Jupiters). One very interesting thing about planets—unlike stars—is that the structure is not just set by the composition; it is also set by the formation history.
2014-10-02
what is the interstellar-medium rest frame?
I spoke with Jeffrey Mei (NYUAD) early in the morning (my time) to discuss his continuum-normalized SDSS spectra of standard stars. We are trying to look for absorption in the spectra that is associated with interstellar medium by regressing the spectra against the Galactic reddening. This is a great project, but has many complicated issues. Not the least is that it is easy to shift the spectra to the stellar rest frame, or even the Solar System barycentric rest frame, but it is hard to shift them to the mean (line-of-sight) interstellar-medium rest frame. I have some ideas, or we could look for blurry features, blurred by the interstellar velocity differences. Maybe Na D will save us?
2014-10-01
Jupiter analogs, and exoplanet music
As with every Wednesday, the highlight was group meeting, which we held (as we do every Wednesday) in the Center for Data Science studio space. We discussed Hattori's search for Jupiter analogs in the Kepler data: The plan is to search with a top-hat function, and then, for the good candidates, do a hypothesis test of top-hat vs saw-tooth vs realistic transit shape. Then do parameter estimation on the ones that prefer the latter. This is a nice structure and highly achievable.
After that, we discussed sonification of the Kepler data with Brian McFee (NYU) and also his tempogram method for looking at beat tracks in music (yes, music). We have some ideas about how these things might be related! At the end of group meeting, we worked on Foreman-Mackey's and Wang's AAS abstracts, both about calibrating out stochastic variability in Kepler light-curves to improve exoplanet search.
2014-09-29
comparing data-driven and theory-driven models
I gave the brown-bag talk in the Center for Cosmology and Particle Physics at lunch-time today. I talked about The Cannon, Ness and Rix and my data-driven model of stellar spectra. I also used the talk as an opportunity to talk about machine learning and data science in the CCPP. Various good ideas came up from the audience. One is that we ought to be able to synthesize, with our data-driven model, the theory-driven model spectra that the APOGEE team uses to do stellar parameter estimation. That would be a great idea; it would help identify where our models and the theory diverge; it might even point to improvements both for The Cannon and for the APOGEE pipelines.
2014-09-28
Gerry Neugebauer
I learned late on Friday that Gerry Neugebauer (Caltech) has died. Gerry was one of the most important scientists in my research life, and in my personal life. He co-advised my PhD thesis (with also Roger Blandford and Judy Cohen); we spent many nights together at the Keck and Palomar Observatories, and many lunches together with Tom Soifer and Keith Matthews at the Athenaeum (the Caltech faculty club).
In my potted history (apologies in advance for errors), Gerry was one of the first people (with Bob Leighton) to point an infrared telescope at the sky; he found far more sources bright in the infrared than anyone seriously expected. This started infrared astronomy. In time, he became the PI of the NASA IRAS mission, which has been one of the highest-impact (and incredibly high in impact-per-dollar) astronomical missions in NASA history. The IRAS data are still the primary basis for many important results and tools in astronomy, including galaxy clustering, infrared background, ultra-luminous galaxies, young stars, and the dust maps.
To a new graduate student at Caltech, Gerry was intimidating: He was gruff, opinionated, and never wrong (as far as I could tell). But if you broke through that very thin veneer of scary, he was the most loving, caring, thoughtful advisor a student could want. He patiently taught me why I should love (not hate) magnitudes and relative measurements. He showed me how a telescope worked by having me observe at Palomar at his side. He showed me how to test our imaging-data uncertainties, both theoretically and observationally, to make sure we weren't making mistakes. (He taught me to call them "uncertainties" not "errors"!) He helped me develop observing strategies and data-analysis strategies that minimize the effects of detector "memory" and non-linearities. He enjoyed data analysis so much, on one of our projects he insisted that he do the data analysis, so long as I (the graduate student) would be willing to write the paper! Uncharacteristically for then or now, he could run his group so efficiently that many of his students designed, built, and operated an astronomical instrument, from soup to nuts, in a few years of PhD! He had strong opinions about how to run a scientific project, how to write up the results, and even about how to typeset numbers. I obey these positions strictly now in all my projects.
Reading this back, it doesn't capture what I really want to say, which is that Gerry spent a huge fraction of his immense intellectual capability on students, postdocs, and others new to science. He cared immensely about mentoring. From working with Gerry I realized that if you want to propagate great ideas into astronomy, you do it not just by writing papers and giving seminars: You do it by mentoring well new generations of scientists who will, in turn, pass it on in their own work and their own students. Many of the world's best infrared astronomers are directly or indirectly a product of Gerry's wonderful mentoring. I was immensely privileged to get some of that!
[I am also the author of Gerry's only erratum ever in the scientific literature. Gerry was a bit scary the day we figured out that error!]
2014-09-26
interstellar bands; PSF dictionaries
Gail Zasowski (JHU) gave an absolutely great talk today, about diffuse interstellar bands in the APOGEE spectra and their possible use as tools for mapping the interstellar medium and measuring the kinematics of the Milky Way. Her talk also made it very clear what a huge advance APOGEE is over previous surveys: There are APOGEE stars in the mid-plane of the disk on the other side of the bulge! She showed lots of beautiful data and some results that just scratch the surface of what can be learned about the interstellar medium with stellar spectra.
In CampHogg group meeting in the morning, we realized we can reformulate Vakili's work on the point-spread function in SDSS and LSST so that he never has to interpolate the data (to, for example, centroid the stars properly). We can always shift the models, never the data. We also realized that we don't need to build a PCA or KL basis for the PSF representation; we can use a dictionary and learn the dictionary elements along with the PSF. This is an exciting realization; it almost ensures that we have to beat the existing methods for accuracy and flexibility. Also interesting: The linear algebra we wrote down permits us to make use of "convolutional methods" and also permits us to represent the PSF at pixel resolutions higher than the data (super-resolution).
2014-09-25
overlapping stars, stellar training sets
On the phone with Schölkopf, Wang, Foreman-Mackey, and I tried to understand how it is that we can fit some insanely variable stars in the Kepler data using other stars, when the variability seems so specific to each star. In one case we investigated, it turned out that the crazy variability of one star (below) was perfectly matched by the variability of another, brighter star. What gives? It turns out that the two stars overlap on the detector, so their footprints actually share pixels! The shared variability is caused by the situation that they are being photometered through overlapping apertures. We also learned that some stars in Kepler have been assigned non-contiguous apertures.
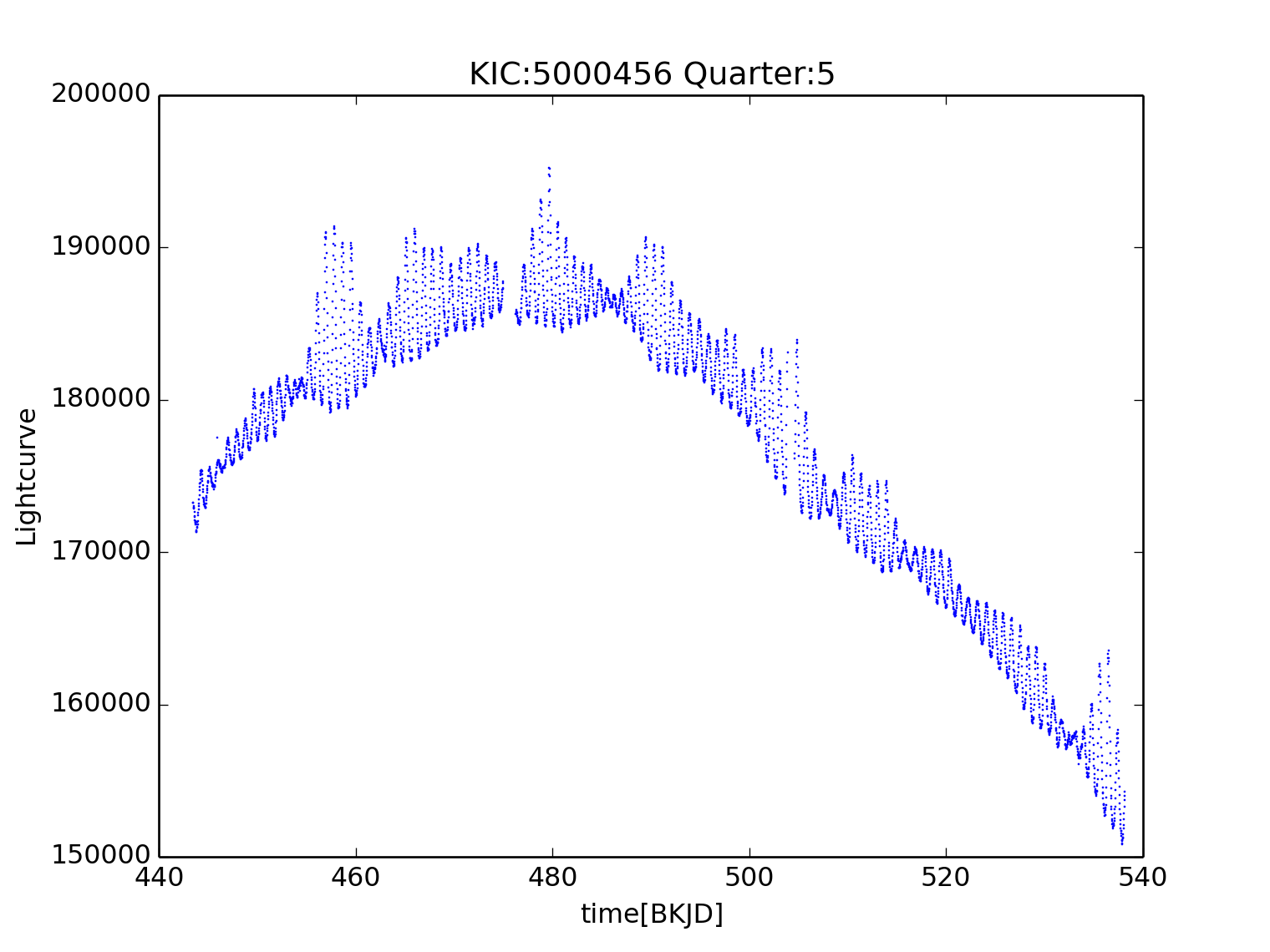
Late in the day, Gail Zasowski (JHU) showed up. I explained in detail The Cannon—Ness, Rix, and my label-transfer code for stellar parameter estimation. She had many questions about our training set, both because it is too large (it contains some obviously wrong entries) and too small (it doesn't nearly cover all kinds of stars at all metallicities).
2014-09-24
deep learning and exoplanet transits
At group meeting, Foreman-Mackey and Wang showed recent results on calibration of K2 and Kepler data, respectively, and Malz showed some SDSS spectra of the night sky. After group meeting, Elizabeth Lamm (NYU) came to ask about possible Data Science capstone projects. We pitched a project on finding exoplanets with Gaia data and another on finding exoplanet transits with deep learning! The latter project was based on Foreman-Mackey's realization that everything that makes convolutional networks great for finding kittens in video also makes them great for finding transits in variable-star light-curves. Bring it on!
2014-09-23
half full or half empty?
Interestingly (to me, anyway), as I have been raving in this space about how awesome it is that Ness and I can transfer stellar parameter labels from a small set of "standard stars" to a huge set of APOGEE stars using a data driven model, Rix (who is one of the authors of the method) has been seeing our results as requiring some spin or adjustment in order to be impressive to the stellar parameter community. I see his point: What impresses me is that we get good structure in the label (stellar parameter) space and we do very well where the data overlap the training sample. What concerns Rix is that many of our labels are clearly wrong or distorted, especially where we don't have good coverage in the training sample. We discussed ways to modify our method or our display of the output to make both points in a responsible way.
Late in the day, Foreman-Mackey and I discussed NYU's high-performance computing hardware and environment with Stratos Efstathiadis (NYU), who said he would look into increasing our disk-usage limits. Operating on the entire Kepler data set inside the compute center turns out to be hard, not because the data set is large, but rather because it is composed of so many tiny files. This is a problem, apparently, for distributed storage systems. We discussed also the future of high-performance computing in the era of Data Science.
2014-09-22
making black holes from gravitons!
I am paying for a week of hacking in Seattle with some days of not research back here in New York City. The one research highlight of the day was Gia Dvali (NYU) telling us at lunch about his work on black holes as information processing machines. Along the way, he described the thought experiment of constructing a black hole by concentrating enormous numbers of gravitons in a small volume. Apparently this thought experiment, as simple as it sounds, justifies the famous black-hole entropy result. I was surprised! Now I am wondering what it would take, physically, to make this experiment happen. Like could you do this with a real phased array of gravitational radiation sources?
2014-09-19
AstroData Hack Week, day 5
Today was Ivezic (UW) day. He gave some great lectures on useful methods from his new textbook. He was very nice on the subject of Extreme Deconvolution, which is the method Bovy, Roweis, and I developed a few years ago. He showed that it rocks on the SDSS stars. Late in the day, I met with Ivezic, Juric, and Vanderplas to discuss our ideas for Gaia "Zero-Day Exploits". We have to be ready! One great idea is to use the existing data we have (for example, PanSTARRS and SDSS), and the existing dynamical models we have of the Milky Way, to make specific predictions for every star in the Gaia catalog. Some of these predictions might be quite specific. I vowed to write one of my short data-analysis documents on this.
In the hack session, Foreman-Mackey generalized our K2 PSF model to give it more freedom, and then we wrote down our hyper-parameters, a hacky objective, and started experimental coding to find the best place to be working. By the wrap-up, he had found some pretty good settings.
The wrap-up session on Friday was deeply impressive and humbling! For more information, check out the HackPad (tm) index.
[ps. Next year this meeting will be called AstroHackWeek and it may happen in NYC. Watch this space.]
2014-09-18
AstroData Hack Week, day 4
Today began with Bloom (UCB) talking about supervised methods. He put in a big plug for Random Forest, of course! Two things I liked about his talk: One, he emphasized the difficulties and time spent munging the data, identifying features, imputing, and so on. Two, he put in some philosophy at the end, to the effect that you don't have to understand every detail of these methods; much better to partner with someone who does. Then the intellectual load on data scientists is not out of control. For Bloom, data science is inherently collaborative. I don't perfectly agree, but I would agree that it works very, very well when it is collaborative. Back on the data munging point: Essentially all of the basic supervised methods presume that your "features" (inputs) are noise-free and non-missing. That's bad for us in general.
Based on Bloom's talk I came up with many hacks related to Random Forest. A few examples are the following: Visualize the content and internals of a Random Forest, and its use as a hacky generative model of the data. Figure out a clever experimental sequence to determine conditional feature importances, which is a combinatorial problem in general. Write a basic open-source RF code and get some community to fastify it. Figure out if there is any way to extend RF to handle noisy input features.
I didn't do any of these hacks! We insisted on pair-coding today among the hackers, and this was a good idea; I partnered with Foreman-Mackey and he showed me what he has done for K2 photometry. It is giving some strange results—it is using the enormous flat-field freedom we are giving it to fix all its ills—we thought about ways to test what is going wrong and how to give other parts of the model relevant freedom.
2014-09-17
AstroData Hack Week, day 3
I gave the Bayesian inference lectures in the morning, and spent time chatting in the afternoon. In my lectures, I argued strongly for passing forward probabilistic answers, not just regularized estimators. In particular, many methods that are called "Bayesian" are just regularized optimizations! The key ideas of Bayes are that you can marginalize out nuisances and properly propagate uncertainties. Those are important ideas and both get lost if you are just optimizing a posterior pdf.
2014-09-16
AstroData Hack Week, day 2
The day started with Huppenkothen (Amsterdam) and I meeting at a café to discuss what we were going to talk about in the tutorial part of the day. We quickly got derailed to talking about replacing periodograms and auto-correlation functions with Gaussian Processes for finding and measuring quasi-periodic signals in stars and x-ray binaries. We described the simplest possible project and vowed to give it a shot when she arrives at NYU in two months. Immediately following this conversation, we each talked for more than an hour about classical statistics. I focused on the value of standard, frequentist methods for getting fast answers that are reliable, easy to interpret, and well understood. I emphasized the value of having a likelihood function!
In the hack session, I spoke with Eilers (MPIA) and Hennawi (MPIA) about measuring absorption by the intergalactic medium in quasars subject to noisy (and correlated) continuum estimation. Foreman-Mackey explained to me that our failures on K2 the previous night were caused by the inflexibility of the (dumb) PSF model hitting the flexibility of the (totally unconstrained) flat-field. I discussed Gibbs sampling for a simple hierarchical inference with Sick (Queens). And I went through agonizing rounds of good-ideas-turned-bad on classifying pixels in Earth imaging data with Kapadia (Mapbox). On the latter, what is the simplest way to do clustering in the space of pixel histograms?
The research day ended with a discussion of Spectro-Perfectionism (Bolton and Schlegel) with Byler (UW). I told her about the long conversations among Roweis, Bolton, and me many years ago (late 2009) about this. We decided to do a close reading of it (the paper) tomorrow.
2014-09-15
AstroData Hack Week, day 1
On my way to Seattle, I wrote up a two-page document about inferring the velocity distribution when you only get (perhaps noisy, perhaps censored) measurements of v sin i. When I arrived at the AstroData Hack Week, I learned that Foreman-Mackey and Price-Whelan had both come to the same conclusion that this would be a valuable and achievable hack for the week. Price-Whelan and I spent hacking time specifying the project better.
That said, Foreman-Mackey got excited about doing a good job on K2 point-source photometry. We talked out the components of such a model and tried to find the simplest possible version of the project, which Foreman-Mackey wants to approach by building a full, parameterized, physical model of the point-spread function, the spacecraft Euler angles, and the flat-field. Late in the day (at the bar) we found out that our first shot at this model is going badly off the rails: The flat-field and the point-spread function are degenerate (somewhat or totally?) in the naive model we have right now. Simple fixes didn't work.
2014-09-12
GRB beaming, classifying stars
Andy Fruchter (STScI) gave the astrophysics seminar, on gamma-ray bursts and their host galaxies. He showed Modjaz's (and others) results on the metallicities of "broad-line type IIc" supernovae, which show that the ones associated with gamma-ray bursts are in much lower-metallicity environments than those not associated. I always react to this result by pointing out that this ought to put a very strong constraint on GRB beaming, because (if there is beaming) there ought to be "off-axis" bursts that we don't see as GRBs, but that we do see as a BLIIc. Both Fruchter and Modjaz claimed that the numbers make the constraint uninteresting, but I am surprised: The result is incredibly strong.
In group meeting, Fadely showed evidence that he can make a generative model of the colors and morphologies (think: angular sizes, or compactnesses) of faint, compact sources in the SDSS imaging data. That is, he can build a flexible model (using the "extreme deconvolution" method) that permits him to predict the compactness of a source given a noisy measurement of its five-band spectral energy distribution. This shows great promise to evolve into a non-parametric, model-free (that is: free of stellar or galaxy models) method for separating stars from galaxies in multi-band imaging. The cool thing is he might be able to create a data-driven star–galaxy classification system without training on any actual star or galaxy labels.
2014-09-11
single-example learning
I pitched projects to new graduate students in the Physics and Data Science programs today; hopefully some will stick. Late in the day, I took out new Data Science Fellow Brenden Lake (NYU) for a beer, along with Brian McFee (NYU) and Foreman-Mackey. We discussed many things, but we were blown away by Lake's experiments on single-instance learning: Can a machine learn to identify or generate a class of objects from seeing only a single example? Humans are great at this but machines are not. He showed us comparisons between his best machines and experimental subjects found on the Mechanical Turk. His machines don't do badly!
2014-09-10
crazy diversity of stars; cosmological anomalies
At CampHogg group meeting (in the new NYU Center for Data Science space!), Sanderson (Columbia) talked about her work on finding structure through unsupervised clustering methods, and Price-Whelan talked about chaotic orbits and the effect of chaos on the streams in the Milky Way. Dun Wang blew us all away by showing us the amazing diversity of Kepler light-curves that go into his effective model of stellar and telescope variability. Even in a completely random set of a hundred light-curves you get eclipsing binaries, exoplanet transits, multiple-mode coherent pulsations, incoherent pulsations, and lots of other crazy variability. We marveled at the range of things used as "features" in his model.
At lunch (with surprise, secret visitor and Nobel Laureate Brian Schmidt), I had a long conversation with Matt Kleban (NYU), following my conversation from yesterday with D'Amico. We veered onto the question of anomalies: Just as there are anomalies in the CMB, there are probably also anomalies in the large-scale structure, but no-one really knows how to look for them. We should figure out and look! Also, each anomaly known in the CMB should make a prediction for an anomaly visible (or maybe not) in the large-scale structure. That would make for a valuable research program.
2014-09-09
searching in the space of observables
In the early morning, Ness and I talked by phone about The Cannon (or maybe The Jump; guess the source of the name!), our method for providing stellar parameter labels to stars without using stellar models. We talked about possibly putting priors in the label space; this might regularize the results towards plausible values when the data are ambiguous. That's for paper 2, not paper 1. She has drafted an email to the APOGEE-2 collaboration about our current status, and we talked about next steps.
In the late morning, I spoke with Guido D'Amico (NYU) about future projects in cosmology that I am interested in thinking about. One class of projects involves searching for new kinds of observables (think: large-scale structure mixed-order statistics and the like) that are tuned to have maximum sensitivity to the cosmological parameters of interest. I feel like there is some kind of data-science-y approach to this, given the incredible simulations currently on the market.
2014-09-08
CDS space
Does it count as research when I work on the NYU Center for Data Science space planning? Probably not, but I spent a good fraction of the day analyzing plans and then discussing with the architects working to create schematics for the new space. We want a great diversity of offices, shared spaces (meeting rooms, offices, and carrels), and open space (studio space and lounge and cafe space). We want our space plans to be robust to our uncertainties about how people will want to use the space.
2014-09-07
writing
Hidden away in a cabin off the grid, I made writing progress on my project with Ness to make data-driven spectral models, and on my project to use hot planets as clocks for timing experiments. On the latter, I figured out some useful things about expected signal-to-noise and why there is a huge difference between checking local clock rates and looking at global, time-variable timing residuals away from a periodic model.
2014-09-05
single transits, group meeting, robot DJs
The day started with a discussion with So Hattori about finding single transits in the Kepler data. We did some research and it seems like there may be no clear sample in the published literature, let alone any planet inference based on them. So we are headed in that direction. In group meeting, Foreman-Mackey told us about his approach to exoplanet search, Goodman told us about his approach to sampling that uses (rather than discards) the rejected likelihood calls (in the limit that they are expensive), and Vakili told us about probabilistic PSF modeling. On the latter, we had requests that he do something more like train and test.
A fraction of the group had lunch with Brian McFee (NYU), the new Data Science Fellow. McFee works on music, from a data analysis perspective. His past research was on song selection and augmenting or replacing collaborative filtering. His present research is on beat matching. So with the two put together he might have a full robot DJ. I have work for that robot!
2014-09-03
calibration and search
A phone call between Wang, Foreman-Mackey, Schölkopf, and me started the day, and a conversation between Foreman-Mackey and me ended the day, both on the subject of modeling the structured noise in the Kepler data and the impact of such methods on exoplanet search. In the morning conversation, we discussed the relative value of using other stars as predictors for the target star (because co-variability of stars encodes telescope variability) compared to using the target star itself, but shifted in time (because the past and future behavior of the star can predict the present state of the star). Wang has a good system for exploring this at the pixel level. We gave him some final tasks before we reduce our scope and write a paper about it.
In the afternoon conversation, we looked at Foreman-Mackey's heuristic "information criteria" that he is using for exoplanet search in the Kepler data. By the end of the day, his search scalar included such interesting components as the following: Each proposed exoplanet period and phase is compared not just to a null model, but to a model in which there is a periodic signal but with time-varying amplitude (which would be a false positive). Each peak in the search scalar is vetoed if it shares transits with other, higher peaks (to rule out period aliasing and transit–artifact pairings). A function of period is subtracted out, to model the baseline created by noise (which is a non-trivial function of period). Everything looks promising for exoplanet candidate generation.
2014-09-02
psf interpolation
Vakili and I spoke about his probabilistic interpolation of the point-spread function in imaging using Gaussian Processes and a basis derived from principal components. The LSST atmospheric PSF looks very stable, according to Vakili's image simulations (which use the LSST code), so I asked for shorter exposures to see more variability. We talked about the point that there might be PSF variations that are fast in time but slow in focal-plane position (from the atmosphere) and others that might be slow in time but fast in focal-plane position (from the optics) and maybe hybrid situations (if, say, the optics have some low-dimensional but fast variability). All these things could be captured by a sophisticated model.
2014-09-01
exoplanet search
Back when we were in Tübingen, Foreman-Mackey promised Schölkopf that he would find some new exoplanets by September 1. That's today! He failed, although he has some very impressive results recovering some known systems that are supposed to be hard to find. That is, it looks like existing systems are found at higher significance for us than for other groups, so we ought to be able to find some lower-significance systems. The key technology (we think) is our insanely flexible noise model for stellar (and Kepler) variability, that uses a Gaussian process not just of time, but of hundreds of other-star lightcurves. Foreman-Mackey and I talked extensively about this today, but we are still many days away from discoveries. We are bound to announce our discoveries on twitter (tm). Bound in the sense of "obligated". Let's see if we have the fortitude to do that!
2014-08-30
detecting clock drifts
I continued working on "hot Jupiters as clocks", building a system to find clock drifts. I find that the precision with which a drift is detected is slightly less than I found for the precision of the clock phase or offset; this makes sense, because any drift model has multiple parameters. It took me a while to get good sampling to work (with emcee, and a sensible burn-in schedule) but it now seems to. I can now start searching for signals. Heather Knutson (Caltech) gave me a couple of suggestions.
2014-08-28
exoclocks: period and phase precision
It is back to the non-research grind now, but that didn't stop me from stealing a couple hours to work on Foreman-Mackey and my "stellar clocks" project. My question was: How precisely can you phase up or measure the time using a transiting hot Jupiter. That is, how good is a hot Jupiter as a clock? If good, we could use it for all kinds of fun things.
I started with fake data. As my loyal reader knows, I am against using fake data; all it provides is a functional test of your code! But over the years I have come around: After all, it provides a functional test of your code! It also permits you to check your results with absolutely known input assumptions; that is, it provides sanity checking on your estimates of (in this case) the precision you expect.
I find, with extremely optimistic assumptions (about the period, depth, duration, ingress time, gravitational dynamics, and, of course, photon noise), that a high-quality transiting hot Jupiter in Kepler normal-cadence data could, over the lifetime of the now-over Kepler mission, provide a time standard that is good to a few tenths of a second. This agrees with the order-of-magnitude calculation I had made myself earlier and suggests that we might be able to do some timing experiments.
2014-08-27
words on a plane
While in transit back to NYC, I worked on writing more about Ness and my quadratic-in-tags model for stellar spectra in our manuscript, and she got the quadratic model to work from end to end. It looks extremely promising.
2014-08-26
data science, data-driven models
Today was my last day in Heidelberg for 2014. Sad day! I spent most of my research time working with Ness on our data-driven models of stars. We got the code to go from first-order (linear) linear fitting to second-order (quadratic) linear fitting, and vowed that we would never go to third order! We will use a Gaussian Process before we do that. We discussed outliers—both outlier stars and outlier pixels—and how to deal with them while still retaining computational tractability, good probability of convergence to a good optimum (or good sampling prospects), and the likelihood principle. We don't have a good solution yet, although I have ideas in my old document on fitting a line. We also discussed next steps on the path to paper 1, including especially making figures that demonstrate the properties and qualities of the individual-wavelength least-square fits we are doing.
At the end of the day, I was noticing that this project is a perfect example of the new discipline of "Data Science", about which I am supposed to have opinions: It was an idea we both had, but I didn't know enough about the spectroscopy, and Ness didn't know enough about the methods available. Neither of us could have done this project without the collaboration (nor without significant amounts of computation).
2014-08-25
debugging
After a few days of vacation, I got back to working on data-driven stellar models with Ness. We spent some time today debugging issues with the tagging of new objects; behavior is mysterious.
2014-08-20
stars in galaxies; data-driven metallicities
MPIA Milky-Way group meeting was excellent today. Ross Fadely talked about our star–galaxy separation projects and showed some very encouraging signs that a supervised regression system to predict stellarity using the object colors might work. We are training not on the labels (star or galaxy) but on the morphological inputs that are used to make those labels. Nicholas Martin (Strasbourg) showed incredible maps of the outskirts of M31 split by metallicity. He is building the maps with a proper forward model of the data. They are gorgeous. He is also experimenting with showing the results of the MCMC sampling as a noisy movie. Cool. Greg Stinson (MPIA) showed both some incredible movies of simulations, and also some interesting results on the delay time distribution for Type Ia SNe. It appears that the direct measurements of the delay time distribution from stellar populations and the inferences of the delay times from chemical abundances might be in conflict. We argued about possible resolutions.
Late in the day, Ness and I closed the loop on the data-driven APOGEE spectral modeling: We used our data-driven model to "learn" the tags (temperature, logg, and metallicity) for a new star. We cheated—we tagged a star that was used in the training—but (a) we rocked it with accurate tag recovery, and (b) we can do cross-validation as a more conservative test. It was an exciting moment.
2014-08-19
the lamentations of radio astronomy
Malte Kuhlmann (MPI-IS), Rix, and I had a great, long meeting with MPIA radio astronomers Simon Bihr, Carl Ferkinhoff, and Laura Zschaechner, to discuss how radio astronomy works and what issues could benefit from some new technology coming from probabilistic inference or machine learning. We located a few points of great interest. One is the problem of flagging data affected by RFI, where it is a time-consuming task but nonetheless there is now a huge quantity of well flagged data to "learn" from. Great place for supervised classification. A second area is calibration of phase delays: The calibrator stars are used to get calibration estimates, which are interpolated to the science data. This interpolation is neither probabilistic nor uses calibration information latent in the science data nor knows about spatial nor time coherence. I am thinking Gaussian Processes, as my loyal reader can imagine. A third area is, of course, scene reconstruction, which is Kuhlmann's current project. Everyone recognizes the great value of CLEAN but also its limitations: It doesn't propagate noise, it contains many heuristically set parameters, and it doesn't optimize a well-specified scalar objective.
In other news, Rix, Ness and I figured out, with our data-driven model of APOGEE spectra, how to label new spectra, and how to identify the most relevant spectral regions to use as metallicity "indices". We outlined paper one and put the code and outline up on github (tm).
2014-08-18
sparse image priors for radio interferometry
Malte Kuhlmann (MPI-IS) arrived in Heidelberg today. We looked at his results on reconstructing astronomical scenes from radio interferometry data. He has done both optimization with a regularized likelihood, and full sampling in image space using hybrid Monte Carlo. He is using an "L1" regularization, which is equivalent to a biexponential prior probability in pixel amplitudes. He finds that he can do a good job of reproducing the input scenes for fake data, when he samples, but that the optimization takes you to a strange place. This is a consequence of the point that in a very high dimensional space, with non-Gaussian priors, the posterior mode an be very far from the posterior mean. We discussed noise propagation for interferometry and also the advantages that a sampling system has over the conventional heuristic methods (such as CLEAN).
Melissa Ness (MPIA) and I worked on adding intrinsic scatter into her model of APOGEE spectra. We need the intrinsic scatter to be understood when we turn her model around and use it to label new spectra for which we don't know the temperature and metallicity. The results we got looked pretty bad, so we have work to do tomorrow.
2014-08-15
causal pixel modeling, day 4
Foreman-Mackey re-started exoplanet search (in Kepler) last night, and on the train home from Tübingen we looked at some of the early false positives. We realized that the way we are constructing the search leads to some non-trivial issues: For performance reasons, we slide a single "box" model across the lightcurve once, and then we do search by linking together likelihood increments from the one-d box slide in periodic groups. The issue is: When we slide the box, we permit the box to take any transit depth, so the system can link together in a periodic group a set of transit-like excursions that all have different depths. A true exoplanet transit signal has a (nearly) fixed depth.
The solution (and this brings back memories of GALEX) is to combine the periodic box results both with and without a constraint that the depths must be identical. The identical model must beat the non-identical model for something to make the search cut. The one complexity is that we have to penalize model complexity: The likelihood of the non-uniform depths model is always better at maximum, because it has much more freedom.
2014-08-14
causal pixel modeling, day 3
After talking to Muandet (MPI-IS) and Lopez-Paz (MPI-IS) about false-positive classification using supervised methods yesterday, Foreman-Mackey sent them some lightcurve information from injected exoplanets and from some random locations, just to give them an idea of what to expect when we start a search for exoplanets in earnest. They classified like the wind. Meanwhile, Schölkopf, Foreman-Mackey, and I discussed the problem of exoplanet search.
The point of our de-trending or modeling of stellar + s/c variability is to make search more effective, and (later) characterization more accurate. Since search is the object, we should optimize with search in mind. We encouraged Foreman-Mackey to start up the search in earnest. One insane thing we realized as we got this started was that the Gaussian-Process-based likelihood function we are using (which uses a Gaussian Process in time or with only one feature, time, as input) can be generalized (in the way described yesterday) to incorporate thousands of other stars as features, with almost no performance hit. That is, we can do the stellar and exoplanet transit modeling simultaneously, even when the stellar model uses information from thousands of other stars! This realization set Foreman-Mackey coding.
With Wang we investigated the possibility that we could do our pixel-level modeling of the imaging data not one Kepler quarter at a time but instead one Kepler month at a time (the data break naturally into months at the DSN data download interruptions). It appears that we can: The full quarter doesn't have that much information in it relevant to short predictions than the month. This potentially speeds up the code by a large factor, because most aspect of our inferences scale up with the size of the data. This is super-true for non-parametric inferences (as with GPs) but also with parametric inferences, both because of internal dot products and also because the bigger the data set the bigger (usually) cross-validation says your feature list should be.
2014-08-13
causal pixel modeling, day 2
In re-reading yesterday's post, I found it strange to hear myself say that the model was over-fitting stellar variability and then we decided to make the model far more flexible! Today we decided that we don't yet have the technology (well, perhaps not the patience, since we want to detect exoplanets asap) to fully separate stellar variability from spacecraft-induced issues, or at least we would have to do something that pooled much more data to do it—we wouldn't be able to work on one light-curve at a time. So we de-scoped to exoplanet science and decided that we would try to fit out everything except the exoplanet transits. This is not unlike what others are doing, except that we are trying to be extremely principled about not letting information in the data about any exoplanet transits "leak" into our modeling of the variability of the light-curve. We are doing this with a censoring or a train-and-test framework.
Because we decided to eradicate all variability—spacecraft and stellar—we had Wang work on auto-regressive models, in which the past and future of the star is used to predict the present of the star. The first results are promising. We also had Foreman-Mackey put all the other stars into the Gaussian Process predictions we are making. This means we are are doing Gaussian Process regression and prediction with thousands of ambient dimensions (features). That seems insane to me, but Schölkopf insists that it will work—being non-parametric, GPs scale in complexity with the number of data points, not the number or size of the features. I will believe it when I see it. The curse of dimensionality and all that!
In the afternoon, we had discussions with Krik Muandet (MPI-IS) and David Lopez-Paz (MPI-IS) about false-positive classification for exoplanet search using supervised methods and a discussion with Michael Hirsch (MPI-IS) about non-parametric models for the imaging PSF. More on the former tomorrow, I very much hope!
2014-08-12
causal pixel modeling, day 1
Today Foreman-Mackey and I arrived in Tübingen to work with Schölkopf. On arrival, we got Dun Wang on the phone, because our trip to MPI-IS is designed to make huge progress on Wang's recalibration of the Kepler satellite detector pixels, using the variations that are found in common across stars. The way Schölkopf likes to say it is that we are capitalizing on the causal structure of the problem: If stars (or, really, pixels illuminated by stars) co-vary it must be because of the telescope, since the stars are causally disconnected. The goal of our work on this is to increase the sensitivity of the satellite to exoplanet transits.
We opened the day with two questions: The first was about why, despite this causal argument, we seem to be able to over-fit or fit out stellar variability. We are being careful with the data (using a train-and-test framework) to ensure that no information about the short-term variability of the star near any putative transit is leaking into the training of the predictive model. My position is that it is because our set of prediction stars might span the full basis of anything a star can do. We are using thousands of stars as features!
The second question was about why, in our residuals, there seems to be some trace of the spacecraft variability. We don't know that for sure, but just at an intuitive visual level it looks like the fitting process is not only not removing the spacecraft, but actually increasing the calibration "noise". We started Wang on tests of various hypotheses, and put Foreman-Mackey on trying models that are far more flexible than Wang's purely linear model.